Japanese (Keynote speech / invited lecture)
Keynote speech
“Challenge of artificial intelligence to transform society”
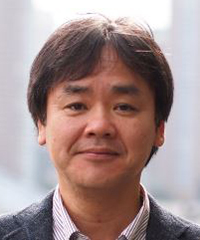
Dr. Naohiko Uramoto
(President of JSAI / Chief Digital Technology Scientist, Mitsubishi Chemical Holdings)
Research and development of Artificial Intelligence (AI) and its applications have been rapidly spreading into society. AI technology and services are now applied to various industrial fields, transforming our industry and society themselves. On the other hand, when AI will be utilized in more complicated and critical situations, we will face not only technical issues but also social and ethical issues. I will cover some discussion points for encouraging the sound growth of our world powered by AI. In this presentation, I will outline the history of AI and the current status, and will discuss on what is the best path we should proceed.
Invited lecture 1
Understanding “Artificial Intelligence”
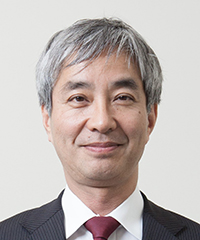
Dr. Hiroshi Maruyama
(Preferred Networks, Inc. Fellow)
“Artificial Intelligence” is an academic discipline; for example, “Artificial Intelligence” in Japan Society for Artificial Intelligence clearly refers to the fields of research. However, the term also used to refer to a system applying technologies derived from this discipline, and it is the source of many confusions, evoking low-precision arguments. In this presentation, we review the history of AI research, point out the possibilities and limitations of statistical machine learning and mathematical optimization which are at the focus of many of current research, and discuss their implications to our future society.
Invited lecture 2
“Explain Yourself – A Semantic Stack for Artificial Intelligence”
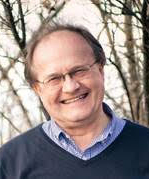
Prof. Randy Goebel
(Professor of Computing Science at the University of Alberta, Canada, and co-founder of the Alberta Machine Intelligence Institute (AMII))
Artificial Intelligence is the pursuit of the science of intelligence. The journey includes everything from formal reasoning, high performance game playing, natural language understanding, and computer vision. Each AI experimental domain is littered along a spectrum of scientific explainability, all the way from high-performance but opaque predictive models, to multi-scale causal models. While the current AI pandemic is preoccupied with human intelligence and primitive unexplainable learning methods, the science of AI requires what all other science requires: accurate explainable causal models. The presentation introduces a sketch of a semantic stack model, which attempts to provide a framework for both scientific understanding and implementation of intelligent systems. A key idea is that intelligence should include an ability to model, predict, and explain application domains, which, for example, would transform purely performance-oriented systems into instructors as well.